A Guide to Implementing Predictive Analytics in Healthcare
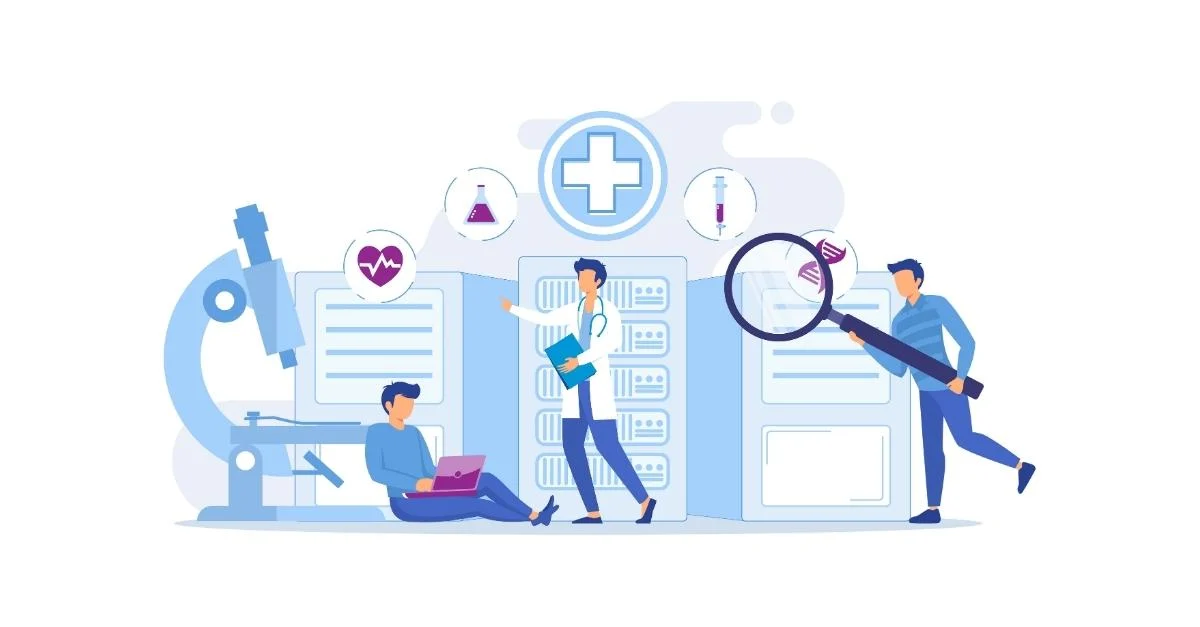
Imagine a world where doctors can predict diseases before symptoms appear, hospitals can prevent patient readmissions, and healthcare providers can optimise resources based on real-time data. Sounds futuristic, right? Well, with predictive analytics, this is already happening!
Predictive analytics is transforming healthcare by using data, statistical algorithms, and machine learning techniques to identify future risks and opportunities. But how can healthcare organisations implement it effectively? Let’s break it down in a simple, engaging way.
What is Predictive Analytics in Healthcare?
Predictive analytics involves using historical and real-time data to forecast potential medical outcomes. Instead of reacting to health issues, doctors and hospitals can prevent them.
For example, if a hospital analyses patient data and finds that certain individuals are at high risk of heart disease based on their history and lifestyle, it can proactively recommend lifestyle changes or early interventions.
Why Does Healthcare Need Predictive Analytics?
The healthcare industry is overwhelmed with data. From electronic health records (EHRs) to insurance claims, wearable devices, and clinical research, data is being generated every second. However, without a smart system to analyse this data, it remains underutilised.
Here’s why predictive analytics is a game-changer:
Early Disease Detection – Helps doctors identify diseases before they become severe.
Reduced Hospital Readmissions – Predicts patients likely to return after discharge and provides preventive care.
Optimised Hospital Operations – Manages staff, bed availability, and resources efficiently.
Personalised Treatment Plans – Uses patient history to suggest the best treatment strategies.
Fraud Detection – Identifies anomalies in insurance claims to prevent fraud.
How to Implement Predictive Analytics in Healthcare
Now that we understand its importance, let’s explore how healthcare providers can successfully implement predictive analytics.
1. Define Your Goals and Use Cases
Before diving in, ask yourself:
What problem do we want to solve?
Are we focusing on reducing hospital readmissions, detecting diseases early, or improving patient experiences?
Example: A hospital struggling with high readmission rates might use predictive analytics to identify at-risk patients and provide extra post-discharge support.
2. Collect and Integrate the Right Data
Predictive analytics is only as good as the data it uses. Healthcare organisations must collect clean, structured, and relevant data from multiple sources, such as:
Electronic Health Records (EHRs)
Medical imaging and lab results
Patient demographics and lifestyle information
Wearable health devices (smartwatches, fitness trackers)
Insurance and billing records
Ensuring data accuracy and consistency is crucial. If the data is incomplete or incorrect, predictions will be unreliable.
3. Use Advanced Machine Learning Algorithms
Predictive analytics relies on AI-driven models that process vast amounts of data and detect patterns. Common techniques include:
Regression Analysis – Identifies relationships between variables (e.g., how smoking increases the risk of lung disease).
Neural Networks – Mimic human brain functions to make complex predictions.
Natural Language Processing (NLP) – Extracts useful information from unstructured medical records.
Healthcare providers can either build custom AI models in-house or use pre-built solutions from AI-driven healthcare platforms.
4. Ensure Data Security and Compliance
Since healthcare data is sensitive, implementing strict data security measures is essential. Predictive analytics solutions must comply with regulations such as:
HIPAA (Health Insurance Portability and Accountability Act) – Ensures patient data privacy in the U.S.
GDPR (General Data Protection Regulation) – Protects patient information in Europe.
POPIA (Protection of Personal Information Act) – Governs data protection in South Africa.
To maintain trust, healthcare providers should use encryption, access controls, and regular security audits.
5. Train Healthcare Professionals and Stakeholders
Predictive analytics is powerful, but human expertise is still essential. Doctors, nurses, and hospital administrators should be trained to:
Interpret AI-generated insights.
Make data-driven clinical decisions.
Recognise potential biases in predictions.
For example, an AI system might predict a high risk of diabetes for a patient, but a doctor needs to confirm it with additional medical tests before prescribing treatment.
6. Monitor and Improve Predictive Models
Predictive analytics is not a one-time setup—it evolves. Hospitals and clinics must continuously monitor their models to ensure they remain accurate and effective.
Regular audits – Check if predictions match real-world outcomes.
Algorithm updates – Incorporate new medical research and technologies.
User feedback – Improve AI systems based on doctor and patient experiences.
Real-Life Examples of Predictive Analytics in Healthcare
Case Study 1: Cleveland Clinic – Used predictive analytics to identify patients at high risk of heart failure, leading to early interventions and fewer hospitalisations.
Case Study 2: Kaiser Permanente – Developed an AI-driven model to predict sepsis in ICU patients, reducing mortality rates by 25%.
Case Study 3: Google’s DeepMind – Created an AI system that analyses eye scans to detect diseases like diabetic retinopathy and age-related macular degeneration.
These examples prove that predictive analytics is not just a buzzword—it’s saving lives!
Challenges in Implementing Predictive Analytics in Healthcare
Despite its benefits, implementing predictive analytics comes with challenges:
Data Privacy Concerns – Patients worry about how their health data is used.
High Implementation Costs – Advanced AI models require significant investment.
Resistance to Change – Doctors and hospitals may be hesitant to trust AI predictions.
Integration Issues – Many healthcare systems use outdated software that doesn’t easily integrate with AI solutions.
The Future of Predictive Analytics in Healthcare
As technology advances, predictive analytics will become more accurate, accessible, and affordable. Future trends include:
AI-powered drug discovery – Predicting which drugs will work best for specific patients.
Wearable-driven health monitoring – Real-time insights from smartwatches and sensors.
Virtual health assistants – AI chatbots providing instant medical advice.
With the right approach, predictive analytics will continue to revolutionise healthcare, improving patient care, reducing costs, and saving lives.
Conclusion
Predictive analytics is changing the healthcare industry—helping doctors make better decisions, improving patient outcomes, and reducing costs. However, successful implementation requires clear goals, quality data, advanced AI models, security measures, and ongoing improvements.
For healthcare providers looking to integrate predictive analytics into their systems, partnering with a custom software development company in South Africa can ensure a tailored, secure, and efficient solution.
Are you ready to leverage AI-driven predictive analytics in your healthcare practice? Let’s talk!